Diagnostics based Asset Predictive Maintenance
AI & MACHINE LEARNING CASE STUDY – INSIGHTFUL IIOT
Predictive Analytics Solutions
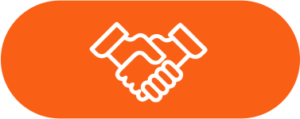
Prelude: In the wake of sensor enabled devices, pervasive digitization, big data platforms and large viable analytics combined with artificial intelligence acceptance took automation diaspora into a different level. Though there are several tools catering to different markets, sizes and verticals, a simple, scalable, actionable and affordable solutions are yet to mature in the present market.
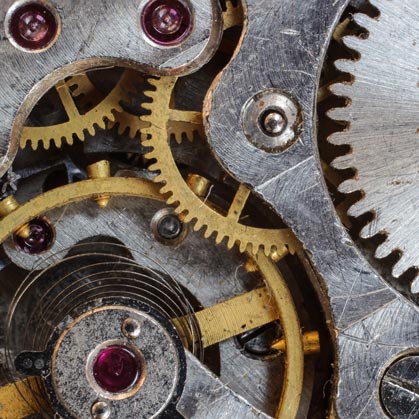
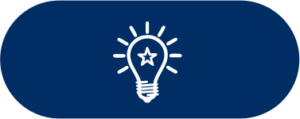
Introduction to Our Solution: Our in-house solution caters to complete data engineering life cycle that begins with cumulating sensor data into data lakes, pre-processing and data preparation, apply ML and AI algorithm wrappers catering to several business cases and showcase the results in interactive dashboards
Solution Highlights
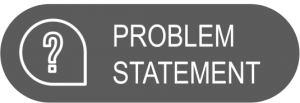
Analyze and diagnose the historical equipment data that has logged several component errors such as overheat, hoist, and break faults. Accordingly, predict and raise the relevant flags and alerts intimating the stakeholders for quick and timely actions
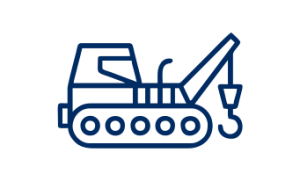
Ingest Data
Cranes operational data gathered from various sensors across geo locations was ingested into AWS storage
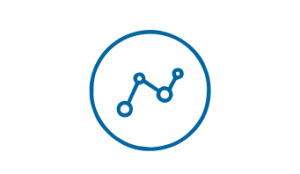
Build the Model
Custom ARIMA models Built toforecast diverse faults across several bins
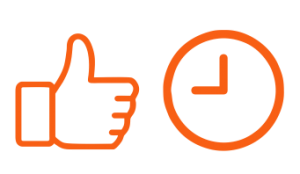
Generate Insights
Predict the imminent faults of different components
The solution used ARIMA algorithm to predict the overall fault volumes as well as location and machinery specific faults
Generated proactive alerts for each machinery highlighting the possible event such as hoist or brake failure
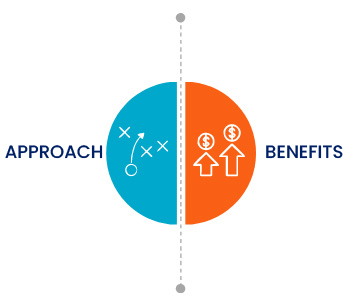
Effective planning of maintenance schedules, shipment of spare parts, and service engineer visits
Reduce maintenance/downtime delays and thus improve overall utilization by 7%
Proactive alerts based on sensitivity and severity (RAG) status
Solution Components & Benefits
CORE TECHNICAL COMPONENTS USED:
- Implementation of supervised and unsupervised clustering models
- Successful implementation of time series models based on ARIMA over Holt Winters for ‘Accurate Forecast’
- Implementation of ‘Survival Models’ based on Cox PH Algorithms
- Overall Simulation was compiled on R and run on AWS Highly Available Cloud Infrastructure
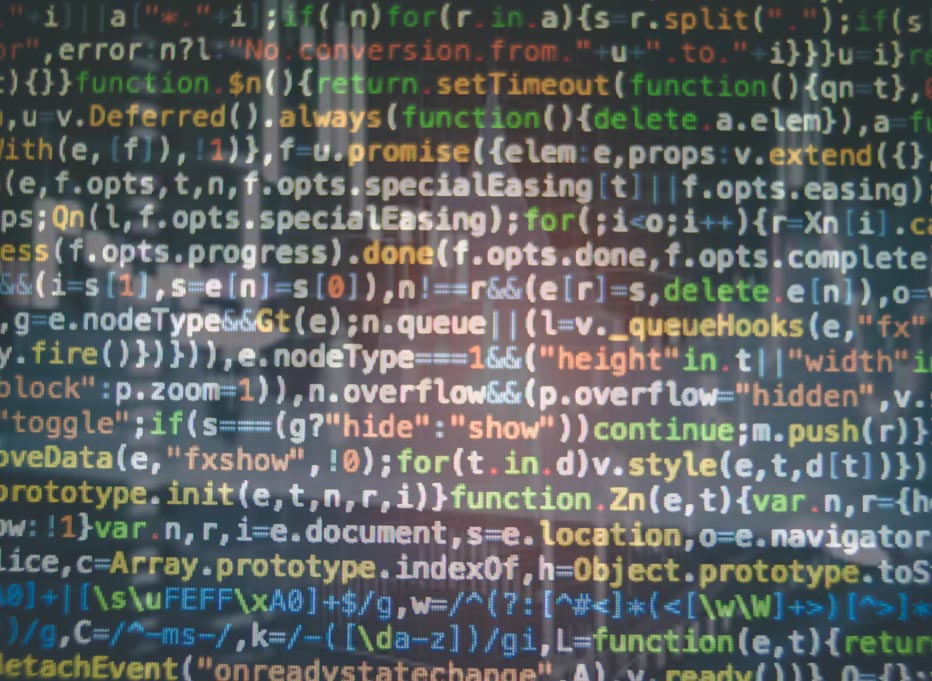
“Solution was developed across 10000+ machinery assets and the predictive models were able to generate accuracies upto 85% in fault prediction diagnostics”